Learning with noisy labels becomes a more and more important topic recently. The reason is that, in the era of big data, datasets are becoming larger and larger. Often, large-scale datasets are infeasible to be annotated accurately due to the cost and time, which naturally brings us cheap datasets with noisy labels. However, the noisy dataset can severely degenerate the performance of machine learning models, especially for the deep neural networks, as they easily memorise and eventually fit label noise. In this project, we are interested to model the noise and then eliminate the side-effect of label noise, i.e., obtaining the optimal classifier defined by the clean data by exploiting the noisy data.
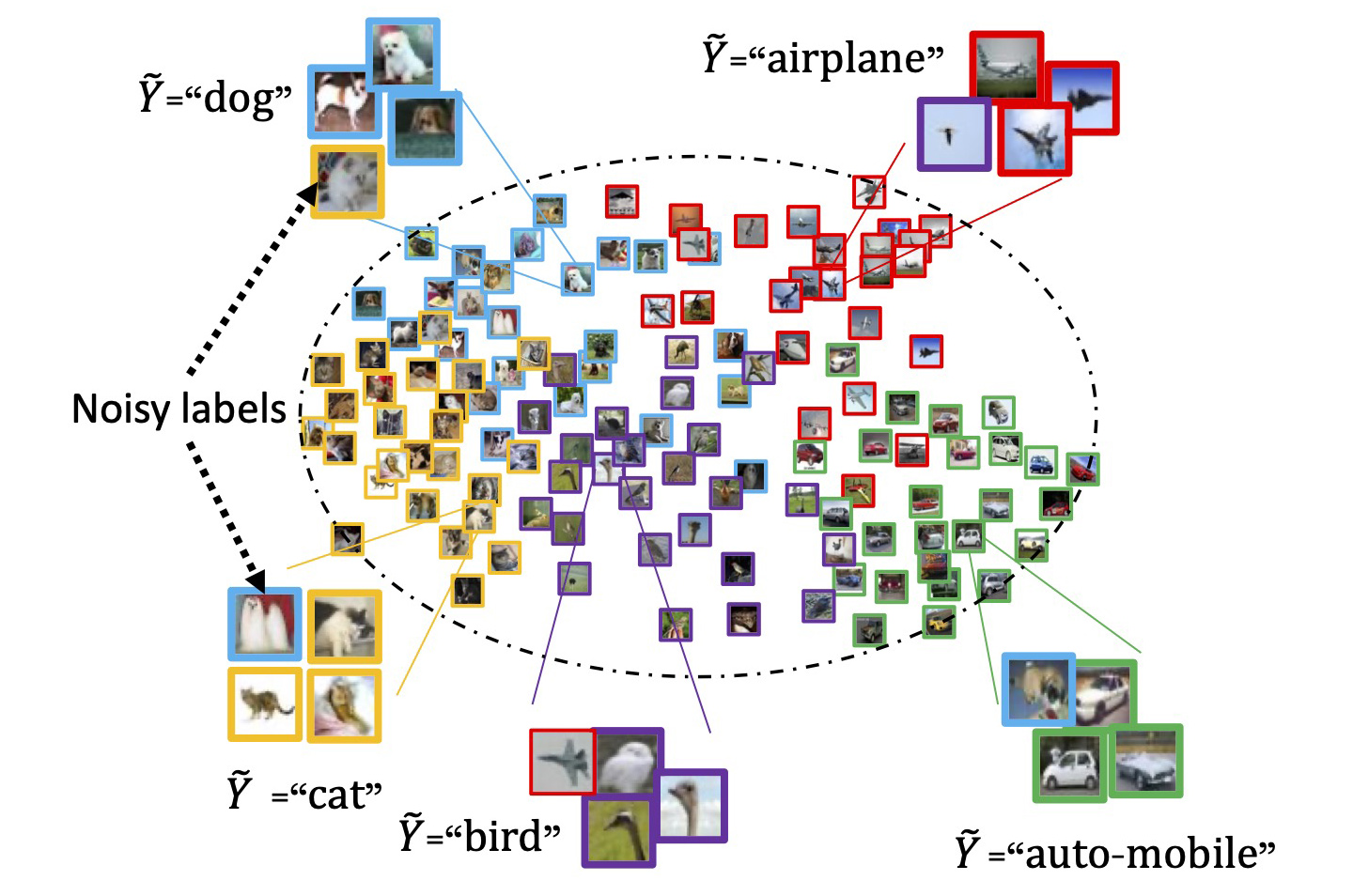