The potential to deliver transformative digital twin technology.
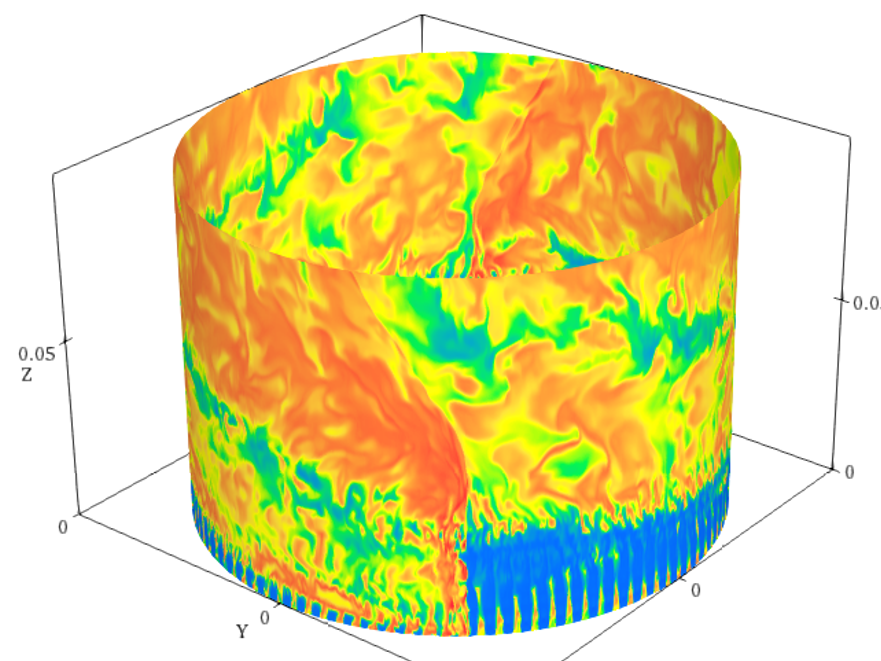
Solving big real-world problems demands an ambitious approach, drawing not only on our investment in fundamental science but also harnessing the unprecedented powerhouse of data now at our fingertips. The exciting new field that splices these two approaches – data-centric engineering – is also the common element running through each pillar of the Digital Sciences Initiative (DSI), driving investigation and discovery.
At its most aspirational and ambitious, data-centric engineering can produce a ‘digital twin’ – a virtual replica of a sophisticated machine or system that allows real-time decision-making and control. This emerging technology has the potential to revolutionise systems, making them more efficient, cheaper to build, maintain and run, and more sustainable.
The relatively new term ‘data-centric engineering’ might be unfamiliar to some, but the University of Sydney has moved quickly to develop its capacity and is emerging as a world-leader, drawing on decades of excellence in fundamental research across AI, robotics, engineering and machine learning as well as agriculture, defence, manufacturing and imaging.
The Data-centric Engineering Group within the Digital Sciences Initiative brings together over 30 data engineering academics with expertise across fluid and particle dynamics, structures and solid mechanics, and complex artificial and natural systems. This spans areas as diverse as atmospheric plumes, bushfires, biofabrication, drug delivery and energy networks. By applying a data-centric framework and partnering with experts in control and logistics, high performance computing and data-science as well industry, we will accelerate research breakthroughs that can be applied across a number of industries.
By its very nature, data-centric engineering is a silo-buster, depending on an interdisciplinary approach. It draws together the best of the more ‘traditional’ research approach of modelling using physics, mathematics and computational techniques with the trillions of gigabytes of data available to create exciting collaborative possibilities.
The ultimate goal is to produce digital twins, which are sophisticated tools for modelling the real world. Taking data from all available sources and turning it into a virtual replica of a machine or system has transformative implications across the DSI’s fundamental pillars of agriculture, medical devices and imaging, defence, and manufacturing.
The University of Sydney is already collaborating with industry partners to develop digital twin technology that will revolutionise design, enabling highly sophisticated modelling that is cheaper, faster and more effective than anything currently available.
But digital twins have the potential to go much further. Seen as an engineering ‘Holy Grail’, they could potentially model complex environments such as bushfires in real-time to help fight them more effectively. They could create a digital simulation of a complex engine, to help further develop and control the system; or they could enable the remote operation of sophisticated control rooms, whether it be on a naval vessel or remote mine site.
Our data-centric approach to engineering will help deliver on the Federal Government’s strategic research priorities across advanced manufacturing, cybersecurity, transport, energy, environmental change and health, soil and water, and food and resources.
The real-world applications of data-centric engineering are almost without limit, and the University of Sydney has the curiosity, commitment and collaborative power to help bring digital twins into the world.
Our vision is to use a data-centric engineering approach to:
- Create a leading educational and research hub for data-centric engineering, developing a sustainable model for identifying, training and supporting the next generation of data-centric engineers
- Combine traditional engineering and scientific modelling approaches with big data and advanced computing solutions to develop digital twins
- Enable real-time data-driven engineering solutions for a range of sectors spanning energy, defence, manufacturing, construction, mining, emergency services, environmental management and more
- Improve safety and longevity of physical infrastructure, and reduce maintenance costs through real-time remote monitoring via distributed sensor technology and cloud-based computing
- Enable the development of autonomous control rooms for naval ships, mine sites, bushfire emergency response rooms and other settings
- Improve the design of combustion, electric and hybrid engines, along with machines for mineral and chemical processing
- Create complex models drawing on data from sophisticated sensors, capturing real-time data from a variety of sources to enhance design, optimise decision-making, and open new market opportunities.
Explore our research
Weekly discussion group
Download the slides below
Presentation slides

Stochastic probability density function (PDF) models for non-linear mechanics
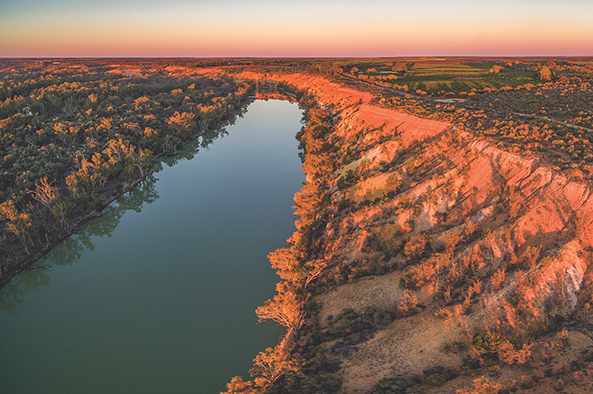
DARE ARC Training Centre
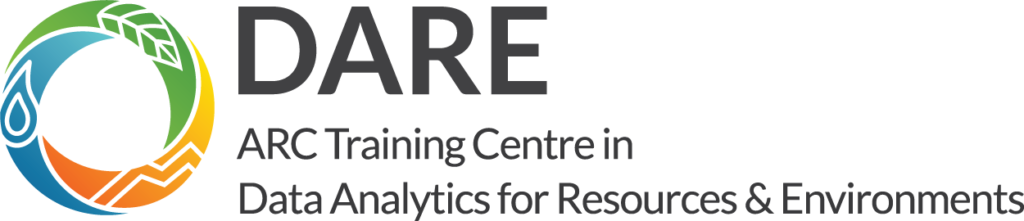
Led by Associate Professor Willem Vervoort, the ARC Industrial Transformation Training Centre in Data Analytics for Resources and Environments (DARE) is developing and delivering the data science skills and tools for Australia’s natural resource industries and managers; to be expert users of data and models; to quantify, explain and understand uncertainty; and to make the best possible evidence-based decisions in exploiting and stewarding the nations’ natural resources and environment.
The DARE Centre is a collaborative research centre led by the University of Sydney in partnership with the University of New South Wales and the University of Western Australia and funded under a five-year grant from the Australian Research Council in partnership with industry and government. DARE’s partner organisations represent some of Australia’s leading organisations directly involved in natural resource use and management.
Core research team
Aerospace, Mechanical and Mechatronic Engineering
- Professor Qing Li
- Professor Ian Manchester
- Associate Professor Matthew Cleary
- Associate Professor Ben Thornber
- Associate Professor Dries Verstraete
- Dr Donald Dansereau
- Dr Michael Groom
- Dr Agisilaos Kourmatzis
Biomedical Engineering
Civil Engineering
- Professor Itai Einav
- Professor Anna Paradowska
- Professor Kim Rasmussen
- Associate Professor Pierre Rognon
- Associate professor Mohammad Saadatfar
- Dr Mani Khezri
- Associate Professor Hao Zhang
Computer Science
Electrical and Information Engineering
- Professor Jian Guo Zhu
- Associate Professor Jin Ma
- Associate Professor Gregor Verbic
- Dr Sinan Li
- Dr Mahyar Shirvanimoghaddam
Life and Environmental Sciences
Physics
Project Management
Phase retrieval and design with automatic differentiation
FSA: Co-designing future high performance systems for efficiency and scalability
Design automation of power electronics: hardware and control
Experiments in multiphase and multiphysics flows
Experimentalist View: Data Processing Methods and Lessons
Data-driven design under uncertainty
Frameworks for DCE with gradient based optimisation
Data-Centric Analysis of Complex Industrial Systems
Seminar replays
The statistical finite element method (statFEM)
Data-centric engineering programme – Prof Mark Girolami, The Alan Turing Institute